Deep learning is one of the most advanced, innovative, and promising technologies of recent years. Its ability to analyze waste data arrays, identify complex patterns, and make data-driven predictions is transforming the way we live and work today. But what is the future of deep learning? Will AI models ever be able to think, ideate and feel like a human?
In this article, we will take a look at machine learning’s future trends, including its potential applications, challenges, and possible impact on society.
Deep Learning Explained Simply
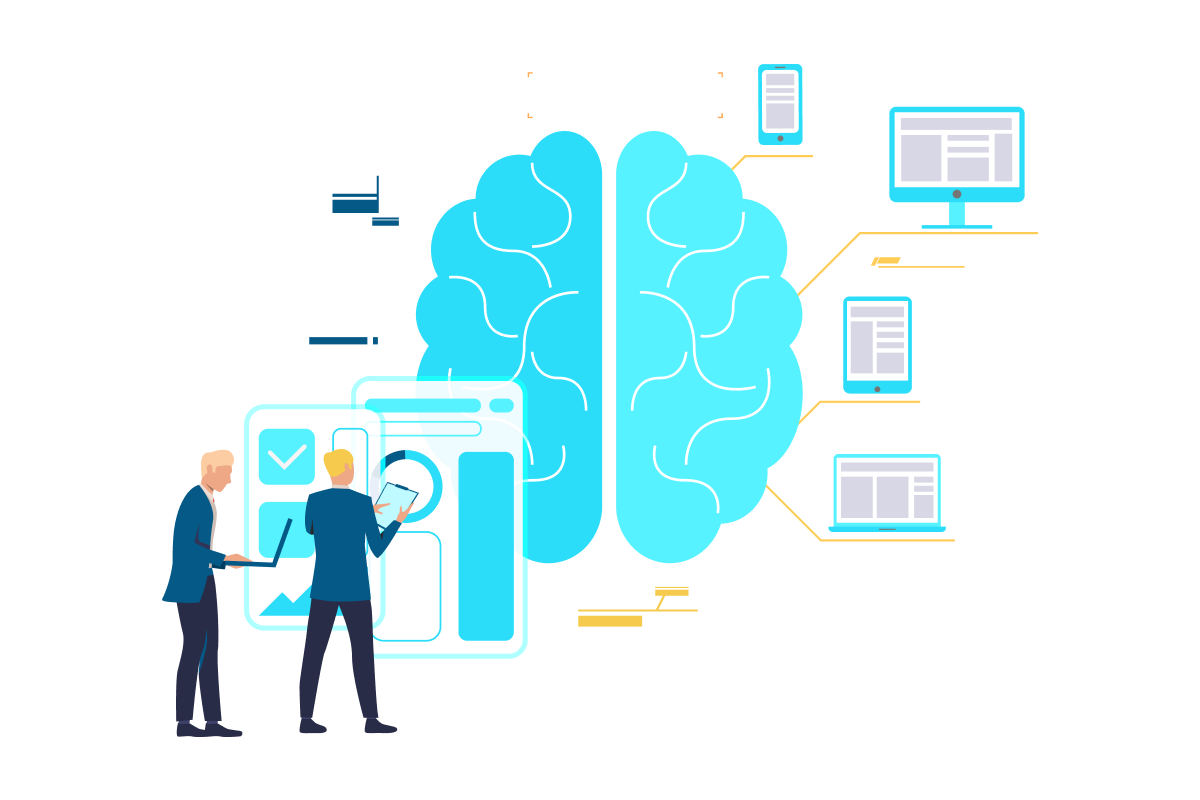
Despite deep learning being a hyped technology, there is still a lot of confusion between it and other AI-related concepts. So, let’s get started from the very beginning. AI or artificial intelligence is an umbrella term that covers rule-based and machine learning algorithms. Machine learning, in turn, can be of three types:
- Supervised,
- Unsupervised,
- Reinforcement.
In addition to these three main types, there are also some hybrid approaches that combine aspects of supervised, unsupervised, and reinforcement learning. Deep learning is one of them.
But what is deep learning precisely? This is an advanced machine learning algorithm that mimics the ways the human brain works. That’s why it is used for such tasks as image recognition, natural language processing, and speech recognition. Even though there is a kind of competition—deep learning vs. neural network—these concepts are sometimes used interchangeably, while neural networks are actually at the heart of deep learning technology.
Now, let’s answer the question of how does deep learning work? To put it simply, it uses artificial neural networks with multiple layers to analyze and learn from data. The main distinctive feature of this technology is that it never stops learning, making new logical connections, and using obtained knowledge for future self-training.
The Most Hyped Deep Learning Model of 2023—ChatGPT
ChatGPT by Open AI needs no introduction as it is the most hyped deep learning model of 2023. As you know, it is an AI language Generative Pre-Trained Transformer that uses unsupervised learning to generate human-like text and interact with the users in a conversational manner.
Immediately after being released, it generated a lot of excitement in the field of deep learning. Overall, the ChatGPT example is likely to be at the heart of all the future transformations neural networks and deep learning will go through in the upcoming years. With its combination of natural language processing capability, unsupervised learning, and possible adoption across industries, ChatGPT is already transforming the next fields:
- Legal services. ChatGPT deep learning technology can help automate legal research, contract review, and other tasks that are time-consuming for lawyers.
- Customer support. Using chatbots as a part of customer service isn’t a new practice, however, ChatGPT can add a human-like touch to the brand’s interactions and learn customer behavior patterns non-stop.
- Finance. ChatGPT also performs well at analyzing financial data, detecting fraud, providing investment advice, and streamlining compliance processes in financial services, such as anti-money laundering (AML) and know-your-customer (KYC) regulations.
- Healthcare. Although deep learning algorithms aren’t going to replace doctors, using this technology can improve patient outcomes significantly. It analyzes vast amounts of patient data, including but not limited to medical history, symptoms, and genetic information, and provides personalized treatment plans.
- Manufacturing. Using deep learning for business in a manufacturing field allows for managing supply chains better, developing predictive and preventive maintenance strategies, and improving quality control.
3 Trends Shaping the Future of Deep Learning
So, while ChatGPT proceeds with transforming major business industries, let’s take an insider look at the future of machine learning and discover the deep learning trends that will shape it.
- Developing models that can learn from smaller data sets. While privacy concerns are growing and collecting data becomes pricier, researchers are investigating how to make a deep learning machine evolve using a smaller amount of data. To solve this challenge, they leverage such techniques as transfer learning, meta-learning, and few-shot learning. This trend is expected to continue in the future, as the need for more efficient and effective deep-learning models grows.
- Developing more interpretable deep learning models. For some fields, such as healthcare and finance, the predictions made by a deep learning network may have critical consequences. That’s why aiming at developing more interpretable models is another trend that will have an impact on the future AI evolution. Now, understanding how the DL model comes to a certain conclusion is difficult, so scientists and researchers will strive to increase transparency and accountability of the interpretable deep learning algorithms.
- Developing more energy-efficient and faster neuromorphic chips. The demand for more efficient and fast-processing deep learning models grows. That’s why reconsidering hardware deep learning architecture is one more trend shaping the future of this innovation. It is expected that new neuromorphic chips that imitate the structure and function of the human brain will be optimized for more advanced types of computation required in a new generation of deep learning.
Critical Areas Where Deep Learning Becomes a Game Changer
The future of machine learning is a double-edged sword that can be both good and bad, depending on how humanity chooses to use it. The world-changing potential of responsible AI is enormous, and when used right, deep learning technologies have the power to make the world a better place. Let’s take a look at the industries in which deep ML is expected to make the most significant impact.
Energy and climate change
According to the World Energy Outlook 2022, now the world is in the middle of an unprecedented energy crisis. Back in 2019, they also researched that global energy consumption is expected to increase by nearly 50% between 2018 and 2050, leading to greenhouse gas emissions and air pollution growing uncontrollably. In this regard, the agency also highlighted the need to reduce energy-related carbon dioxide emissions to net-zero by 2050 in order to limit global warming to 1.5 °C. And this is where deep learning and machine learning can help by enabling more efficient energy systems.
For example, at the University of California, scientists have developed a deep learning model that can predict renewable energy generation from wind and solar sources, allowing grid operators to better manage energy supply and demand. In addition, deep learning models can be pretty effective for climate shaping and change prediction, allowing government authorities and private businesses to take preventive measures in advance.
Hunger and food waste
According to the UN report, 10.7% of the world’s population suffers from hunger. At the same time, one-third of all food produced globally is wasted, which will be sufficient to feed 2 billion people. But how deep learning technology can help with the global problem of hunger and food waste?
To find the answer, it is necessary to understand that waste occurs at all stages of the food supply chain, from production to consumption. The only difference is where, when, and for what reason it happens. While in developing countries (which need food most) it takes place earlier in the supply chain, in developed countries it occurs at a consumer level, with households and restaurants throwing away large amounts of edible food.
Fortunately, machine and deep learning technologies are capable of solving the issue at every stage of food production and distribution by optimizing supply chains, predicting spoilage, classifying and separating waste, or recommending recipes based on ingredients that are about to expire.
Crop yields and water shortage
The global problem of hunger is also directly related to the issue of water shortage and consequently, crop yields. For the agricultural industry, the value created through the use of deep learning exceeds the necessary investment significantly. For example, with the help of agricultural robots that are projected to be largely adopted in the US, farmers will be able to get data-driven insights into their plant’s health and moisture levels.
As for the latter, making data-first decisions about the necessary water supply for each plant is a way to significantly improve crop yields and avoid water overuse. While the use of robots in agriculture is still relatively new, deep learning and computer vision technologies have significant potential to revolutionize the industry.
Recycling
Neural networks and machine learning are becoming wider adopted for improving the recycling process and the accuracy of waste sorting. For instance, DL algorithms can be trained to recognize different types or subtypes of materials, like plastic of different chemical compounds. In addition to waste sorting and identification, improving recycling efficiency, and reducing contamination, deep learning can also help with the development of new recycling technologies.
For example, a deep learning model developed in 2021 can separate waste into three main categories—plastic, paper, and metal—using only a colored PNG of the waste. Since the global society becomes better aware of the need to recycle, there is a high probability that deep learning technology will evolve in response to this demand, making recycling more efficient and cost-effective.
Transportation
The future of deep learning looks even more exciting when it comes to the ways it is expected to change the transportation industry. Being at the heart of self-driving cars, this technology is promising for making roadways significantly safer. For example, Tesla’s self-driving technology could reduce traffic accidents by up to 90%, saving thousands of lives each year.
The adoption of self-driving cars is also expected to have a significant impact on the environment, reducing carbon emissions by up to 60%. Given the self-learning mechanism inside a deep learning model, it would be safe to predict that this technology will work towards improving the accuracy and reliability of self-driving cars year by year.
Conclusion
In this article, we have discovered why machine learning is the future of most industries and how this technology is going to revolutionize them. You are also welcome to change the ways your business operates with advanced artificial intelligence programming services custom-matched to your company’s needs. Our in-house team of ML development experts would be glad to share their latest expertise and help you drive innovation within your organization!
Drop us a line now to book a free call with our AI tech team!